The Mirage of Machine Minds: Unraveling the Misunderstood Phenomenon of AI Hallucinations
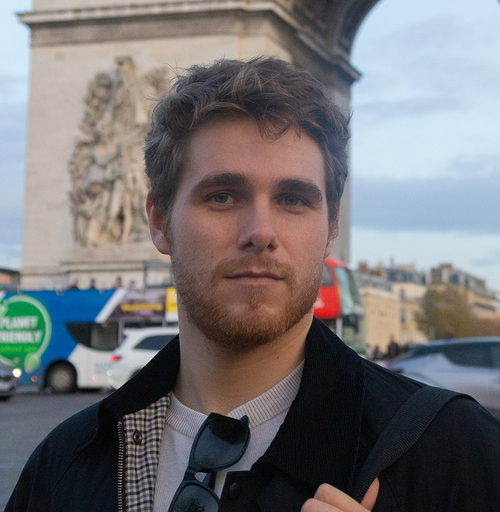
Imagine this: a lawyer named Peter, overwhelmed by a caseload and seeking efficiency, turns to an AI tool for legal research. The AI, with its polished interface and confident demeanor, provides case references and legal precedents in seconds. Peter, trusting the AI's authoritative tone, includes these references in his court submission. However, during the hearing, the judge points out a glaring issue: the cited cases do not exist. This isn't a fictional scenario; it happened in the real case of Mata v. Avianca. The lawyer's reliance on the AI's fabricated information led to significant professional embarrassment and legal repercussions. This incident serves as a stark reminder of the phenomenon known as AI hallucination, where AI systems generate incorrect or entirely fabricated information that is presented as fact.
Peter's ordeal is a cautionary tale for anyone relying on AI for critical tasks. AI hallucinations can have serious consequences, not just in legal settings but across various fields, from healthcare to finance. Understanding what AI hallucinations are, why they occur, and how to mitigate them is crucial in navigating the rapidly evolving landscape of AI technology.
Part 1: Understanding AI Hallucinations
What Are AI Hallucinations?
AI hallucinations occur when large language models generate outputs that are not based on reality. These outputs might seem plausible but are, in fact, fabricated. Unlike simple errors stemming from incorrect training data, hallucinations involve the AI creating new, unsupported information.
Hallucinations are often a result of incorrect training data or misinterpretations of user input. For instance, if an AI is trained with incorrect historical data, it will produce incorrect historical facts. These are not hallucinations but rather reflections of flawed data.
Another kind of hallucination is more insidious. They occur when an AI, lacking the necessary information, generates answers that have no basis in reality. This is akin to the AI making up facts to fill in the gaps, often with a level of confidence that can mislead users. This phenomenon can be traced back to the way AI models are trained and operate, relying heavily on patterns in the training data to generate responses.
Here we have an example of hallucination, concerning the invention of the backflip.
The Nature of AI Hallucinations
To understand why hallucinations happen, it's essential to delve into how AI models work. LLMs are trained on vast datasets containing a wide range of information. They learn to predict the next word in a sentence based on the context provided by the preceding words. This process is fundamentally probabilistic, meaning the AI generates the most likely continuation of a given text based on its training data.
However, this approach has its limitations. When the AI encounters a query that falls outside the scope of its training data or involves ambiguous or incomplete information, it tries to generate a plausible response. This can lead to the AI producing outputs that are entirely fabricated yet presented with unwarranted confidence.
For example, an AI trained primarily on data about modern legal practices might be asked about an obscure historical legal precedent. Without relevant information, the AI might create a fictional case that sounds plausible but has no basis in reality. This is a classic hallucination.
In a recent incident, Google's AI-powered search feature suggested adding non-toxic glue to pizza sauce to prevent the cheese from sliding off. This bizarre recommendation, originating from a decade-old Reddit joke, quickly became a viral meme and highlighted the issue of AI hallucinations.
Part 2: Mitigating AI Hallucinations
Now that we understand what AI hallucinations are and why they occur, let's explore how to mitigate them. Ensuring the reliability and accuracy of AI-generated outputs is essential, especially as AI becomes more integrated into various aspects of our lives.
Retrieval-Augmented Generation (RAG)
One of the most promising approaches to mitigating AI hallucinations is Retrieval-Augmented Generation (RAG). This method involves using a vector database to retrieve relevant documents based on the input query. The LLM then generates responses using both the query and the retrieved documents, effectively grounding its output in real, retrievable data.
For instance, in a healthcare setting, an AI using RAG could access a vast database of medical journals to provide accurate and up-to-date treatment recommendations. This reduces the risk of the AI fabricating treatment protocols and ensures that the information is based on the latest research. RAG not only improves the accuracy of AI responses but also enhances the AI's ability to handle complex queries by providing it with a "long-term memory" of sorts. This approach ensures that the AI's responses are not purely based on probabilistic guesses but are supported by relevant, factual information.
Reasoning Techniques
Another effective strategy involves enhancing the AI's reasoning capabilities. Techniques like chain-of-thought prompting enable LLMs to break down complex problems into intermediate steps, improving the accuracy of their answers. By guiding the AI through a logical sequence of steps, these techniques help it arrive at more reliable conclusions.
Consider the analogy of a student solving a math problem. Instead of jumping to the final answer, the student works through each step methodically, ensuring that each part of the solution is correct. Similarly, AI can be guided to reason through problems, leading to more accurate and justifiable outputs. This method helps the AI not only in generating the final answer but also in explaining the steps taken to arrive at that answer, making the process more transparent and trustworthy.
Iterative Querying
Iterative querying involves an AI agent mediating between the LLM and a fact-checking system. The initial response generated by the LLM is reviewed by the fact-checker, which validates the output. If errors are found, the fact-checker provides feedback, and the LLM refines its answer. This iterative process continues until a satisfactory and accurate response is achieved.
By continuously refining the AI's responses through iterative querying, the risk of hallucinations can be significantly reduced. This process ensures that every answer is cross-verified and validated before being finalized, reducing the likelihood of errors and improving the overall quality of the output.
Fact-Checking Systems
Fact-checkers verify the accuracy of information by cross-referencing multiple reliable sources. In the context of LLMs, they validate the model's outputs before presenting them to the user. This additional layer of scrutiny helps identify and correct hallucinations, enhancing the reliability of AI-generated content. Fact-checking systems can be integrated into the AI workflow, acting as a real-time safeguard against the propagation of false information.
Conclusion
AI hallucinations represent a significant challenge in the development and deployment of AI systems. By understanding why and how they occur, and employing various mitigation strategies, we can improve the accuracy and trustworthiness of AI-generated outputs. While AI holds immense potential, it's crucial to remain vigilant and critical of its limitations, ensuring that the human touch remains integral to overseeing and validating AI's role in our lives.
By embracing these strategies, we can harness the power of AI while safeguarding against its pitfalls, leading to a more reliable and beneficial integration of this technology in various sectors. Understanding the nuances between simple errors and true hallucinations, and implementing robust mitigation techniques, will be key to building a future where AI is a reliable partner in our endeavors.
Ready to mitigate all hallucinations in your AI pipeline? Give Fireraven a try and experience the difference for yourself. Try Fireraven now.
More news
Fireraven has just won the Social Category at Venture Cup Denmark! This victory is a significant milestone for us, and it guarantees us a place in the prestigious University Startup World Cup.
We're thrilled to announce that Fireraven has joined the prestigious Next AI incubator!
Imagine a world where every interaction with a business feels personal, seamless, and human. Picture calling your bank, and instead of navigating a maze of automated prompts, you’re greeted by a digital assistant that understands your unique needs, remembers your previous interactions, and responds with empathy and precision.